Understanding How AI Art Generators Create Stunning Visuals
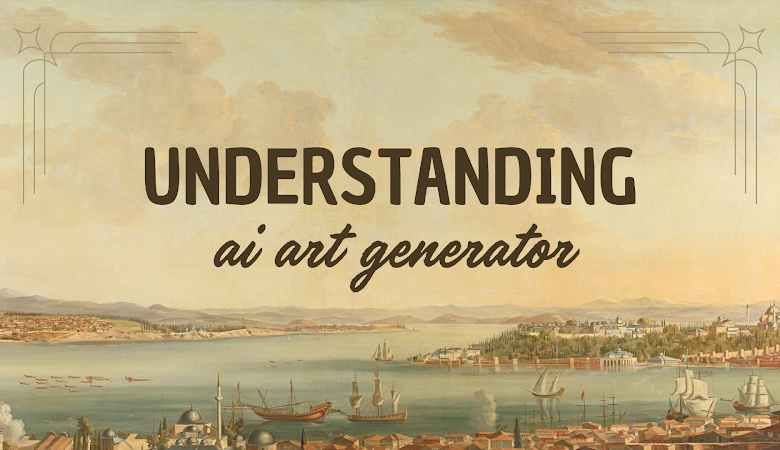
Do you often find yourself thinking about AI, especially when it comes to AI art generation? It’s incredible how you can turn a simple idea into art by just entering the right prompt into an AI tool.
You’ve probably been thrilled when you received the perfect visual content that matched your work aesthetic. Accessing images has never been this easy. While it may seem like magic, it’s actually advanced technology working seamlessly behind the scenes.
No need to puzzle over it anymore. It’s time to address that common question about how AI art generators work. Let’s deepen our understanding of AI art generation today.
You’ve probably come across various art generators and are familiar with how some of them work. Tools like ImagineArt DALL·E, Hotpot, Canva, and Adobe Firefly are just a few examples.
Let’s take a closer look at one such impressive AI art generator: the AI Art Generator by ImagineArt. The results are high-quality and fast, but the process behind them is not simple; it involves a lot of technicalities.
AI tools like ImagineArt are initially trained on vast datasets, consisting of millions of images and texts. A machine can’t distinguish between objects unless it’s properly trained. These tools are fed with data until they can recognize specific objects and the text associated with them.
For example, if you enter the prompt “A standing white horse,” the tool must be able to recognize the horse and its attribute of being white. To do this, the AI art generator relies on a vast dataset that helps it analyze your prompt and generate the desired result.
The AI Process: From Prompts to Images
The ability of machines to analyze prompts and determine the output is powered by technology based on neural networks. A neural network is an algorithm that helps the tool differentiate specific data from vast datasets. It is through this algorithm that tools like ImagineArt can identify a horse from millions of images in the system.
With the help of machine learning algorithms and neural networks, these tools can also recognize additional attributes.
When you mention an apple, only a sensible and perceptive human brain can visualize it. But how can a machine depict an apple when asked? Does it even possess such intelligence? In a way, yes, and that’s why we refer to it as “Artificial Intelligence.”
A machine can possess such intelligence only when it’s trained with multiple algorithms. When you enter a prompt describing a mango tree in an AI art generator, it relies on the knowledge it has gained about what a mango tree looks like. It must be able to differentiate a mango tree from all other trees it has encountered in its training.
The AI then adjusts its processing based on the additional attributes you provide. The AI art generator you’re using has a vast collection of images. It identifies patterns that match your prompt.
To deliver the desired results, an AI art generator must first understand the prompt you enter. To achieve this, AI art tools are trained using text-image pairs. The algorithm is designed to ensure that these tools can correctly interpret and process the text without confusion.
The text you provide is transformed into an image through a process known as Natural Language Processing (NLP). This involves machine learning algorithms that help the system understand human language.
The text-to-image generation process uses deep learning models like Generative Adversarial Networks (GAN) and Variational Autoencoders (VAE) to create images from text.
Improving Results with Better Prompts
The datasets initially fed into the AI are essential for teaching the system to understand the connection between text and visual content. When you enter a prompt, the text signals the system to identify patterns within the datasets, allowing it to generate an image that aligns with your prompt.
It’s important to note that the quality of images generated in text-to-image models depends on several factors:
- Complexity of input text: More detailed prompts often lead to better and more accurate images.
- Quality of training datasets: High-quality datasets help the AI recognize patterns and produce clearer images.
- Architecture of AI models: The design of the AI models impacts their ability to process and generate images effectively.
- Techniques used: Advanced methods like deep learning contribute to producing high-quality results.
AI models like Flux AI and ImagineArt are known for producing high-quality images.
You can always refine your prompt to achieve better images. Being specific about the type of image you want is key. The AI art generator processes your text, and the quality of the generated image depends entirely on your prompt.
So, it’s important to be precise when entering your prompt.
Generative AI Models Behind the Scenes
While each AI art generation tool has unique features that set it apart, the core technology used for AI art generation is largely similar across platforms. Let’s take a look at some basic generative AI models:
- Generative Adversarial Networks (GANs)
- Variational Autoencoders (VAEs)
- Diffusion Models